A short summary of what we know about the brain.
The Ocean Within Us
The story of the brain – or its neurons – begins about 4 billion years ago, with the arrival of the first organism on Earth: the oval-shaped unicellular Paramecium, which still exists today.
On its search for charged particles (atoms with an electric charge, also referred to as ions), which are its food source, the Paramecium swims through vast oceans. It is propelled forwards by the movement of little hairs (called cilia) on its semi-porous membrane. Whenever it bumps into something, the impact opens channels on its membrane, which allows it to process and gain energy from calcium and potassium ions from the surrounding water. This then generates enough energy to power cilia movement, which create a force strong enough to push it away from the obstacle. In this way, the Paramecium then continues its search for ions in its natural environment. This process of being powered by a taking in and pushing out of charged particles is mirrored by what fuels the activity of individual cells in our brain – in this way, the Paramecium can be thought of as being a swimming neuron.
When life got more complex, and multicellular animals with entire nets of nerve cells emerged, they supplied the neuron-clusters in their bodies with charged particles in their blood flow. In a way, we are now carrying the sea and its charged particles in our skulls in the form of a steady blood supply.
However, before neural nets in early multicellular animals began to cluster in such a way to lead to the emergence of the first brain, it is likely that they had their neurons distributed across their entire body with no true neuron-specialisation, as this is the simplest form a neural system can take. This would have worked best for animals with a perfectly symmetrical body, as no part of them would have been more at risk of danger than another, or more likely to encounter food first.
Having a mouth at the front and moving forwards through the world changes this. When the head is the first part of the body to be in contact with both food and danger, the senses – and hence the central nervous system – also need to be in the head. After all, having the senses centralized in this way reduces the length of nerve-wiring relevant signals need to travel which benefits reaction times of the animal to orient its head away from danger, and towards (potential) reward.
Neuron-specialisation
This process, called cephalization, led to more complex neural systems mostly clustered in one central brain, with inter-neurons mediating between stimulus impact and response through relevant pre-processing of the input data (rather than the direct connection between both in the neuron-like Paramecium).
Over time, this process has resulted in the immensely complex human brain with about 90 billion neurons, with over 100 trillion interconnections between them.
Weighing 1.2kg, which is only about 2% of our body weight, the brain consumes roughly 20% of our energy, showing how crucial it is for our functioning. Yet, the neurons themselves have not changed much, and are identical throughout all known species (and almost identical within species, although there are different types of neurons, which vary in morphology and functions).
What does differ across species is not the complexity and diversity of neurons, but their number. The brains of honey bees, the most advanced insects, have about 1 million neurons, for example. Worms, like nematodes, have about 300. The number of neurons as well as their position in the brain is a good indicator for processing power of the organism. For instance, a higher number of neurons in the forebrain indicates greater intelligence, with humans taking the clear lead.
Intelligence
The Anatomy of a Neuron
Aside from a difference in numbers across species and some variation in morphology and function between neuron types within species, all neurons share a number of features: they all have short arms (called dendrites) that perform input functions to the neuron's cell body, and longer arms (axons) that transmit information to its output region (the synapse, which is a pathway between neurons). Here, the neuron's axon interfaces with a dendrite of another neuron.
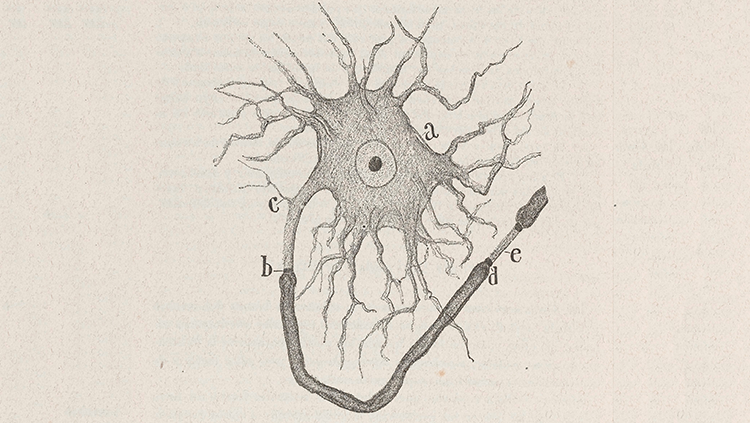
Figure 1: Basic Neuron Structure
A close-up schematic drawing of a neuron by Santiago Ramon y Cajal who – in the early 20th century – first proposed the brain to be a cellular machine. It shows a neuron's cell body (a), its short dendrites (c), long axons axons myelinated in a sheath from (b) to (d), the presynaptic terminal where the neuron interfaces with another neuron (d), and finally the synapse at (e), followed by the postsynaptic terminal of another neuron's axon.
Source: Historical Neuroscience Archives
As a signal arrives at a synapse, it opens up calcium channels at the presynaptic terminal (the output region of a neuron performing an input to another neuron). This causes a presynaptic influx of charged calcium ions. The charge activates proteins that allow for the release of little packets of neurotransmitters (chemicals that transmit information from one neuron to another over the synapse) through the presynaptic membrane, referred to as "synaptic vesicles".
They then drift over the gap from the presynaptic neuron, and bind to the receptors on the postsynaptic terminal. Both neurotransmitters and their receptors have specific shapes, which, like puzzle pieces, have a very precise fit, and then bind together to allow for signal transmission. In this way, neurotransmission is a bit like sending a letter. The sender, the presynaptic neuron, writes a letter to the postsynaptic neuron and the types of neurotransmitters used make up the message of the letter, which tells the recipient neuron what to do.
Once a receptor on the presynaptic terminal of a neuron has been activated, a brief electrical response results, which we call a synaptic potential. The synaptic potential then travels up one of the dendrites to the neuron's cell body. Synaptic potentials can be either positive or negative – depending on the presynaptic neuron and its neurotransmitters. In consequence, they can be either inhibitory or excitatory. Specifically, a synaptic potential will be inhibitory if it makes the overall voltage in the receiving neuron more negative, and excitatory if it makes it more positive. At rest, a neuron has a stable (resting potential) voltage across its membrane of about -70 microvolt (µV), with changes in its charge and consequent activity being a consequence of the summation of all its inhibitory and excitatory potential inputs via connected dendrites.
If the summation of all neuron inputs is positive, it will generate an action potential of +50 µV before returning to its resting potential. The more positive the excitation, the higher the frequency at which the neuron will fire. This is important, as we will explore later, as the firing frequency of a neuron codes for important information. The only limiting factors on the frequency of the neurons' pulse train are their individual refractory periods (the time periods following the generation of an action potential in which the neuron cannot fire), which differ according to their physical attributes.
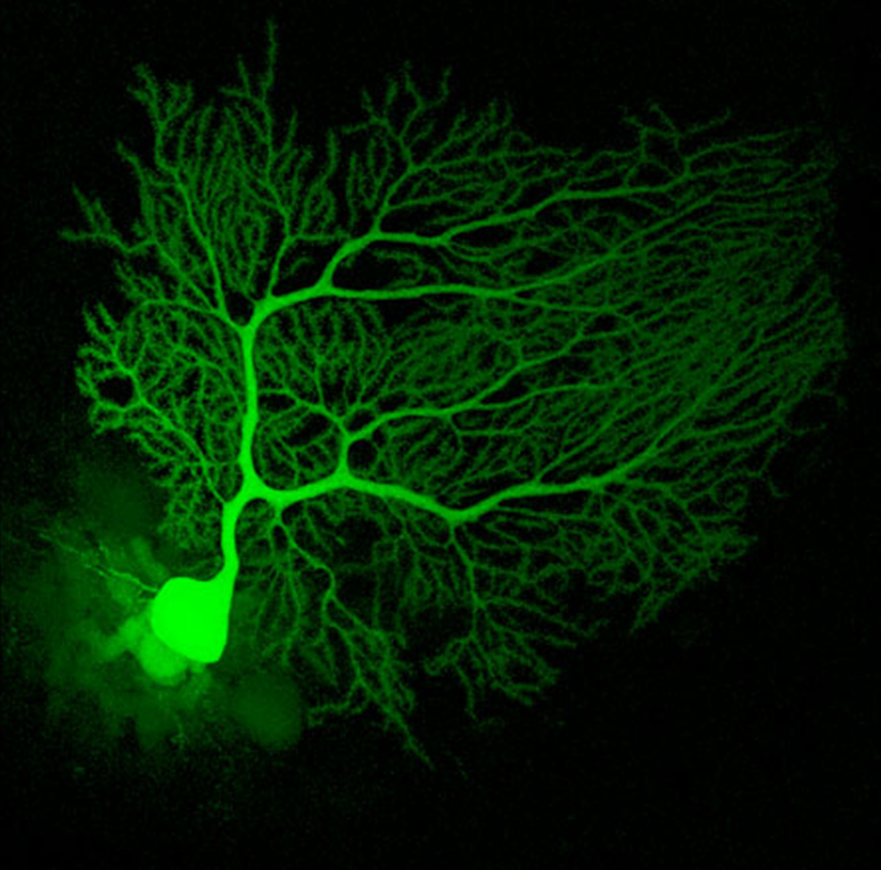
Mouse Cerebellum Neuron
An actual neuron from the cerebellum of a mouse infused with a fluorescent dye called Lucifer Yellow to light it up under a fluorescence microscope.
Source: Neuroscience Research Lab
Neuronal axons, on their own, are terrible electricity conductors, as they easily leak it into surrounding fluids. This is why the brain spends about 80% of its energy on improving their length constant (a measure over which about ⅔ of an electrical signal's amplitude is lost) by boosting the signal the neuron is sending with biological batteries.
Biological Batteries
A neuron has a 50 µV battery facing inward (with the positive pole facing inside the cell body), and a 70 µV battery with the positive pole facing outward the cell body. In the resting state, the outward-facing 70 µV battery is turned on, and the inward-facing 50 µV battery is turned off, causing the resting potential of -70 µV inside the cell body. An action potential comes about when the state of the batteries flips rapidly, which is initiated by a positive voltage-shift across the neuron's membrane.
Both batteries are charged by proteins that pump positively charged ions in opposite directions across the neuron's membrane: sodium ions are pumped out, and potassium ions are pumped in. This creates a charge, as the pumping both exploits and maintains an imbalance between the inside and outside concentration of both ions.
Specifically, there's ten times more sodium outside, and ten times more potassium inside the neuron's cell body. This means that, following the second law of thermodynamics, without the membrane barrier there would be an influx of sodium and an efflux of potassium to reach an equilibrium condition.
Protein molecules – called ion channels – restrict the ion movement, however. Specifically, each neuron has ion channels for potassium and sodium. The potassium channels features activation gates that, when opened, allow for the inflow of potassium into the cell body, switching on the outward-facing 70 µV battery and switching off the inward-facing 50 µV battery. This creates the neurons' -70 µV resting potential.
Neural signal transmission
The kind of synapse described so far is referred to as a close apposition chemical synapse. In their simplest form, they involve an ion channel receptor (also known as an ionotropic receptor) which generate a brief postsynaptic charge after being opened by a neurotransmitter binding. Close apposition chemical synapses are one-way communication channels, with neurotransmitters being sent from presynaptic terminals (which specialise in neurotransmitters synthesis, storage and release) to postsynaptic terminals which recognise and then convert these neurotransmitters into electrical signals. Because of how common this form of communication is, neurotransmitters are also referred to as 'primary messengers' in the brain.
Another form of a close apposition chemical synapse involves indirect metabotropic receptors, which use 'secondary messengers'. On binding, they generate a biochemical rather than an electrical signal, which is mediated by enzymes that synthesise signalling molecules. This gives metabotropic receptors the advantage that they can transmit information directly into the neuron's nucleus, which causes long-term change in gene expression and protein synthesis. As this modulates the strength of the synapse, neural communication via secondary messengers plays an important part in neuroplasticity.
A simpler form of point-to-point communication between neurons happens in the form of direct contact electrical synapses. In contrast to close apposition chemical synapses, the communication can occur in both directions and involve almost no delay, as the electrical signal directly passes through protein pores perforating the membrane of both electrodes. On the other hand, while this rapid signal transfer is great for automatic behaviour, as the neural computations get more complicated, direct electrical communication becomes increasingly limited. This is where chemical communication via primary and second messengers comes in, as this allows for more complex computations.
In any case, no matter the form of neural communication, even with the use of these biological batteries to boost the signal, neurons aren't ideal for conducting electricity. When their speed is compared to the wires we use in computers, which transmit information close to the speed of light, the conduction velocities of the brain – about 120 meters per second at best – seem sluggish.
One way that the brain tries to improve its transmission speeds is by increasing the axon's diameter, as this boosts conductivity. There's a catch, however. To double speed, the axon diameter has to quadruple. Clearly there are limitations to this approach then, as there's a trade-off between signal transmission speeds and how many neurons you'd be willing to sacrifice in order to fit enlarged axons inside a skull of limited size. Still, in some animals this strategy has lead to huge axons. While in humans, axons are rarely bigger than 0.01 mm, in the squid, for example, some reach up to 1mm in diameter, which allows for extremely quick signal transmission and – in this case – equally rapid reaction times in danger situations.
Another way to boost conductivity of axons (leading to a boost in transmission speed) is insulation. In the brain, this is taken care of by myelin (a white insulating sheath around nerve fibres). Myelin wraps around axons in 1mm intervals, interrupted by gaps known as nodes of Ranvier (named after their discoverer Louis-Antoine Ranvier). At these nodes, which expose the axonal membrane, sodium channels give the signal an extra boost. Myelin fulfils a critical function, and when it is damaged, as it is in multiple sclerosis, significant motor and cognitive problems result.
Non-neural communication
Myelin is produced by glial cells (non-neural cells in the central and peripheral nervous system) which, aside from insulating axons, also have a number of other very crucial features for brain function. Glial cells outnumber neurons tenfold (which already implies their importance) and they can take a variety of forms. Microglia, for example, can move around the brain freely, consuming dead cellular debris as they go.
Other types of glial cells can actively modulate neurons and inter-neuron interactions via gliotransmitters at synapses, or by covering or uncovering regions of inter-neuron communication, thereby directing traffic between parts of the brain.
While the inter-neuron communication via gliotransmitters is still restricted to point-to-point communication between synapses, there are other ways for the brain to transmit information. Importantly, neurons can communicate without synapses through the release of freely diffusing messenger molecules like nitric oxide (a gas), which increases blood vessel dilation and hence blood flow. This is important, as the oxygen and glucose carried in the blood powers the brains' operations.
Blood Flow as a Messenger
Even though the total level of blood in the brain stays constant, its flow is diverted dynamically based on what regions of the brain are currently active and hence require more resources. In addition, the blood supply functions as an alternative communication channel between the brain and the body – carrying important information outside of neurons altogether. When endocrine glands, for example, release hormones into the bloodstream, they are carried up into the brain and inform it about the state of bodily functions. It then reacts by depositing hormonal instructions back into the blood supply, which is then distributed throughout the body. Indicating their importance, blood vessels make up about 20% of the brains' volume.
The Structure of the Brain
The interaction between brain and body via hormones already implies that our nervous system is heavily interconnected. Following this, not all our neurons are centralised in the brain, but can also be found in the spinal cord. In fact, the functions of the spinal cord are so fundamental to taking in input from our body, that one could say that the brain practically extends the length of the backbone.
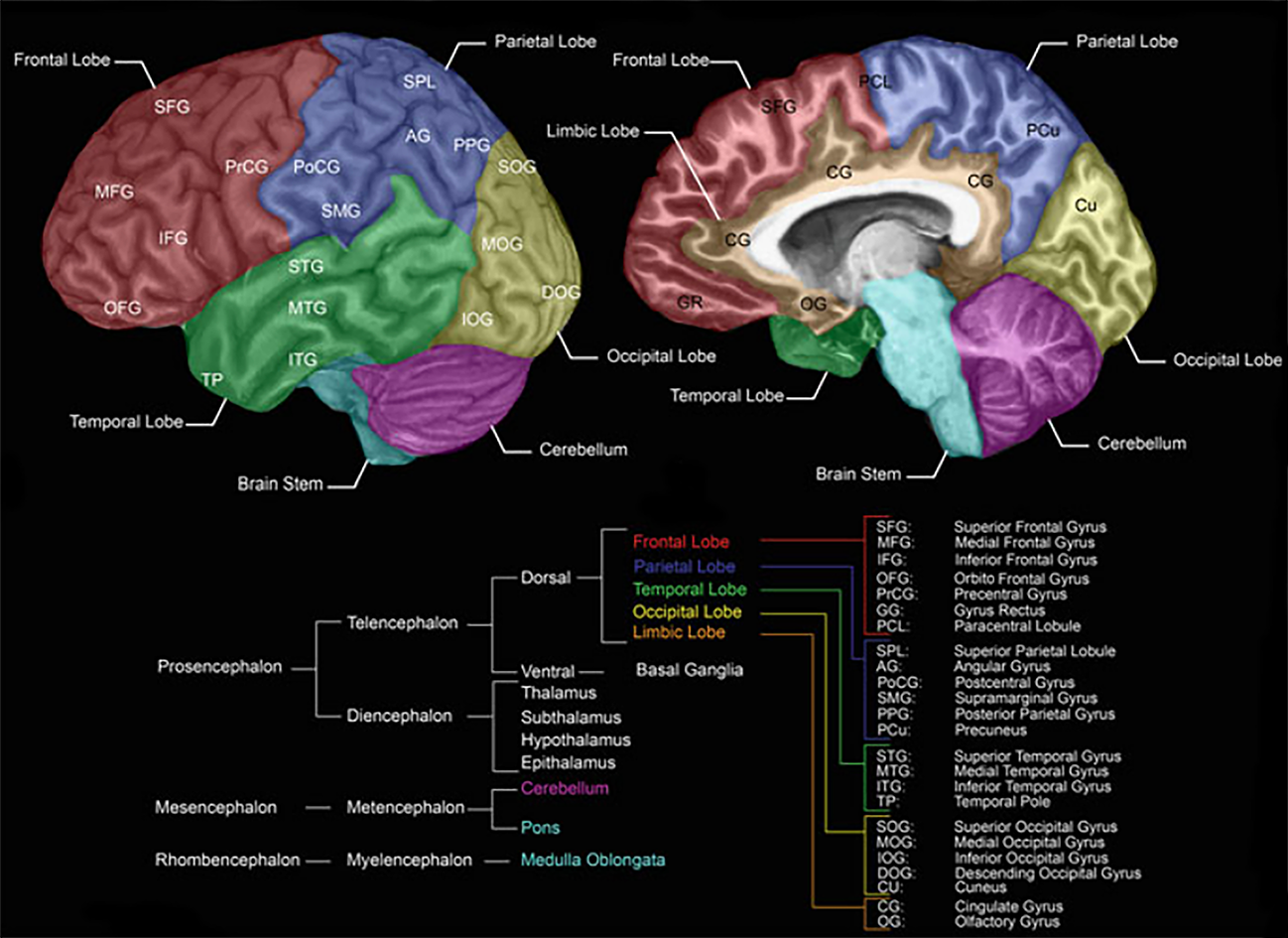
Brain Structure and Regions
Modified from Forstmann B., Keuken M., Alkemade A. (2015) An Introduction to Human Brain Anatomy.
Source: Springer, New York, NY
In the brain, there are three major divisions – the forebrain, midbrain, and the hindbrain – with the latter being connected directly to the spinal cord, making up the central nervous system. As information moves up from the spinal cord over the hindbrain and midbrain to the forebrain, it gets processed and transformed in an increasingly complex manner, ultimately giving rise to cognition.
The spinal cord, just like the brain, contains a central region of grey matter (neuron synapses and cell bodies) surrounded by white matter (myelinated axons) transmitting information up and down the cord. In the spinal cord, outgoing axons of motor neurons connect to the brain, and incoming axons of sensory neurons communicate sensory input to it, with inter-neurons communicating between both pairs.
The connections of the spinal cord run directly to the hindbrain, which regulates automatic bodily functions via the medulla. The cerebellum, which is also part of the hindbrain, then takes care of the execution of body movements, as well as their encoding as movement memories. Finally, the pons in the hindbrain establishes a communication pathway with the midbrain to further process the inputs coming from the spinal cord, as well as to eventually send additional commands down the hierarchy.
From the pons, information travels upward to the midbrain which takes care of basic sensory processing. Specifically, it consists of the substantia nigra (movement sensations), inferior colliculus (hearing sensations), and the superior colliculus (seeing sensations).
At this point, information reaches the forebrain – starting with its evolutionary older components contained in the diencephalon. This part of the forebrain is part of the limbic system (containing the amygdala, hippocampus, basal ganglia, and the cingulate gyrus), which deals with behavioural and emotional responses and plays a central role in learning. It also houses the hypothalamus which deals with homeostatic and circadian mechanisms of the body and brain. For this purpose, the hypothalamus also directly connects to the pituitary gland which sends off hormones into the bloodstream as a means of brain-body communication. Most crucially for our purpose, the diencephalon contains the thalamus, which functions as a relay station for sensory input from the midbrain on its way up to the forebrain.
From the diencephalon, information finally reaches the telencephalon, which is the newest (in evolutionary terms) part of the forebrain and especially big in humans. So big in fact, that it had to be folded in on itself to even fit inside the skull, forming six distinct layers of hierarchical cortical organisation. This part of the brain processes more complex sensory information and acts as a sort of executive centre. For this purpose, it houses the aforementioned basal ganglia, as well as the cerebral cortex, which is the focus of my research.
The cerebral cortex is subdivided into two largely symmetrical hemispheres connected by one million axons of the corpus callosum, and it can be subdivided into four basic areas, with their own respective specialisations. The frontal area of the brain, for example, mostly deals with executive functions. Behind it, in the middle of the brain, we find the parietal area, which contains the somatosensory cortex that maps onto real or imagined physical sensations (via the sensory homunculus) and receives sensory input from the spinal cord over the pathway I've described above. Adjacent to the somatosensory cortex we find the motor cortex which activates for any kind of real or imagined muscle movement and sends generated signals back down to the spinal cord over motor nerves in order to trigger respective movements. Below the parietal area, we find the temporal areas to the side of each hemisphere, which deal with language, memory, real or imagined smell and hearing, while, at the very back of the brain, the occipital area is specialised for vision (again, both real and imagined).
During development, from the initial formation of the brain onwards, connections between neurons are undergoing constant change. Some of those changes are short-term only. An example of short-term changes is the phenomenon of habituation, which describes the observation that continuously firing neurons lessen in responsiveness over time. Practically this can mean that if you give your brain the same stimulus over and over again, its response will be less and less pronounced, meaning you need an increasingly stronger stimulus to keep getting a similarly strong response.
Thankfully, another short-term change in neural wiring provides an easy way out: you can pair an old "habituated" stimulus with a novel one, causing a pronounced neural response (this is referred to as sensitisation or dishabituation). Given frequent manifestation, short-term changes in brain activity patterns could become more permanent. This can mean the formation of new synapses, but from birth onwards most neuroplastic change comes in the form of a changing of synaptic connection strengths. Either, connections become stronger as a consequence of being used a lot – an observation often referred to as Hebbian "neurons that fire together, wire together" potentiation (named after one of its early popularisers Donald Hebb) – or they become weaker via long-term depression of activity, which leads to synaptic pruning, that is, the cutting off of existing connections.
As a majority of brain development consists of nothing but pruning of existing connections, with almost all neurons and their inter-connections developing early on, this also means that, in the case of brain damage, the fully developed brain's ability to grow new neurons through neurogenesis – especially outside the hippocampus – is negligible.
It's likely that the brain's inability to regenerate following brain damage is because of inhibitory molecular signals given off by damaged myelin which prevent regeneration of severed axons. The olfactory system is an exception to this, where a special form of myelin is produced by olfactory ensheathing glial cells and actively promotes neural growth. In fact, when, in 1994, Ramon-Cueto and Nieto-Sampedro extracted some of these glial cells from the olfactory system and implanted them into a patient's damaged spinal cord, severed axons were able to grow back.
It's not clear why central neural pathways have developed responses to prevent this regeneration from happening naturally. In consequence, however, the brain has evolved to be highly plastic. In the case of damage, this means that the remaining parts of the brain are able to carry out lost functions which would be completely impossible in the peripheral nervous system. In fact, a still-developing neural system can shed most of its structure without significant loss in function. This can be seen in some cases of hydrocephalus, where a build-up of fluids in the brain compresses it to a thin neural layer underneath the skull – so much so that in magnetic resonance imaging (MRI) scans the brain almost appears to be missing entirely.
At this point, we have briefly sketched the composition of the brain from single neurons to somewhat fluid modular neural assemblies, its extension down the spinal cord and its non-neural communications. If you want to dive deeper, I recommend my more advanced article on Neural Oscillations, but if you are wondering why you should care at all, read on.
Why Study the Brain
One reason why I think studying the brain is worthwhile is that it will hopefully help our ability to aid people with brain disorders more effectively, restore lost brain functions to those who have sustained brain damage as a consequence of injuries, or augment existing abilities (like intelligence).
In addition, I think that understanding the brain better might teach us a lot about how to more effectively build better artificial minds – both narrow and more general in their intelligence – to make our lives easier.
The investigation of neurons has already inspired the use of artificial neural networks (ANNs) in machine learning, which have proven more effective than many had anticipated. Put simply, ANNs are biology-inspired connectionist systems which, through trial-and-error, change association weights between hierarchically-organised classification nodes, until the output at the highest-order layer has a high-enough classification accuracy.
The ANN algorithms might be further improved by a deeper understanding of how actual neurons differ from simple classification nodes. For example, at least pyramidal neurons in human brains (and possibly neurons more generally) differ from said artificial neurons in the sense that each individual pyramidal neuron itself is effectively behaving like a two-layer ANN. In the first layer, synaptic input arrives at individual sigmoidal nodes (dendrites of the neuron), are then summed up in a second layer node (the neuron's cell body), which performs final threshold.
This allows complex logical operations – like XOR – to be performed in just a single neuron (Poirazi , Brannon and Mel, 2003). XOR ( ) stands for "exclusive or" and is a logical operation which returns a true value only if one input value () or another () is true, but not when both are true at the same time. Formally, this is expressed by . In neurons, this logical operation is performed via a thresholding mechanism, which outputs a weak signal if the input is below the threshold, a stronger signal as it approximates the threshold, and a progressively weaker one as it exceeds the threshold value (Gidon et al., 2020).
Even this doesn't capture the complexity of neural processing adequately, however. Taking the chemical processing at close apposition chemical synapses into account, the computations performed by a single pyramidal neuron are even more complex, and can only be adequately modeled using a deep temporally convolutional network (TCN), with 7 layers and 128 channels (David, Idan and Michael, 2019).
If indeed all neurons have this feature, thinking about the brain as a neural network would be misleading, rather it would be a meta-neural network, which itself consists of billions of individual neural networks. Still, regardless of its complexity, just like the brain benefits from having nested neural networks with many layers, ANNs benefit from adding an increasing number of additional layers – more "neurons" to their network. In fact, these "deep learning" ("deep" due to the depth of involving many layers) algorithms often show that performance in various tasks rises as a function of their increasing complexity.
Another way in which the human brain is distinctly different from traditional ANNs, is its use of non-synaptic inter-neuron communication. One of the most prominent examples of this is how the brain uses nitric oxide to change the flow of blood, glucose, oxygen, and neurotransmitters. GasNet neural networks address this, as they simulate gaseous modulatory neurotransmission inspired by messenger molecules. Generally they achieve a similar performance to traditional ANNs using a simpler architecture with fewer artificial "neurons". When generated via genetic algorithms, for example, the number of generations required is tenfold fewer than for traditional neural nets (e.g. Husbands et al., 1998), which speaks for their higher efficiency.
Genetic Algorithms
Aside from implementing many layers and non-synaptic neural communication, ANNs might benefit from incorporating a form of temporal coding. I'm saying this because, while neurons are often likened to simple logic gates, information between neurons is carried not just in their action potentials, but in their relative rate of firing.
In other words, groups of neurons can communicate not just by sending an on/off signal to each other, but can influence their respective activity by sending on/off signals at specific rhythms, allowing for massively parallel processing between local and global networks. Artificial spiking neural networks are an attempt of leveraging the benefits of this design in algorithmic form, with recent work showing promise that temporal coding in deep spiking ANNs might be significantly (42%) more computationally efficient than traditional deep ANNs, with only very minor (0.5%) trade-offs in accuracy (Zhang et al., 2019).
From this perspective, brain function is not just a consequence of brain anatomy and connectivity, but also of its temporal structure. If true, then this has major implications for both therapeutic/restorative and augmentative applications of neuroscience, as well as for what lessons machine learning researchers can learn from the brain or its constituent neurons. This, to me, is one of the most fascinating areas of neuroscience research, and something I have written about in more detail in my "Neural Oscillations" article.
Sources
David, B., Idan, S. and Michael, L., 2019. Single Cortical Neurons as Deep Artificial Neural Networks. bioRxiv, p.613141.
Forstmann B., Keuken M., Alkemade A. (2015) An Introduction to Human Brain Anatomy. In: Forstmann B., Wagenmakers EJ. (eds) An Introduction to Model-Based Cognitive Neuroscience. Springer, New York, NY
Gidon, A., Zolnik, T., Fidzinski, P., Bolduan, F., Papoutsi, A., Poirazi, P., Holtkamp, M., Vida, I. and Larkum, M. (2020). Dendritic action potentials and computation in human layer 2/3 cortical neurons. Science, 367(6473), pp.83-87.
Husbands, P., Smith, T., Jakobi, N. and O'Shea, M., 1998. Better living through chemistry: Evolving GasNets for robot control. Connection Science, 10(3-4), pp.185-210.
Ramón-Cueto, A. and Nieto-Sampedro, M., 1994. Regeneration into the spinal cord of transected dorsal root axons is promoted by ensheathing glia transplants. Experimental neurology, 127(2), pp.232-244.
Poirazi, P., Brannon, T. and Mel, B.W., 2003. Pyramidal neuron as two-layer neural network. Neuron, 37(6), pp.989-999.
Zhang, L., Zhou, S., Zhi, T., Du, Z. and Chen, Y., 2019, July. Tdsnn: From deep neural networks to deep spike neural networks with temporal-coding. In Proceedings of the AAAI Conference on Artificial Intelligence (Vol. 33, pp. 1319-1326).