Understanding biofeedback technologies...
Control Systems
The term "feedback" is commonly used in engineering to describe control systems operating via their ability to detect changes in the environment of their operations and then to make internal adjustments to keep functions continuously optimized to its demand.
An example of a control system is the thermostat, which uses a temperature sensor to switch heating/cooling devices on/off to maintain – or closely approximate – a set goal temperature.
The body has similar feedback mechanisms, like pupil accommodation for near or far vision or respiration during a heavy workout. Most of these systems are performed automatically and are hence out of conscious control. This is not an issue as long they work normally, but when their automatic adjustments break down or malfunction, we are not usually equipped with restoring their function by just taking over control ourselves.
Biofeedback is a proposed solution to this. It involves taking a real-time measure of whatever bodily process normally not available to awareness, and presenting the measure to the user. The measure could, for example, be represented as a coloured square on a monitor, that gets brighter as the measure gets closer to an ideal set-point, or an audio tone that changes pitch as the state of the user improves. Through this, biofeedback aims to make the user aware of their own physical and psychological processes, with the goal of it helping the body of the user to adjust accordingly – either by providing the user with unconscious reward/punishment signals, or by training them to consciously control previously automatic functions.
Neurofeedback
In 1929, Berger realised that the electrical activity of the brain could be measured from the scalp. This is a consequence of clusters of neurons firing at once – either spontaneously, resulting from a stimulus or as the result of rhythmic oscillations generated by the brain itself. The resulting change in voltage, can then be observed via electroencephalography (EEG) as a peak in the resulting waveform.
One of the first observations of Berger was, that when people closed their eyes, a frequency component close to 10 Hz at the back of the head became more prominent. It also got more pronounced as people grew more relaxed or were drifting off to sleep. He dubbed this the "alpha rhythm", as it was the first frequency range of interest that he catalogued.
Current research explains the function of alpha oscillations as thalamo-cortical inhibitors of neural activity. In other words, alpha acts as a brake on cognition or brain function. This is one of the ways in which the brain optimises its own functioning: as the processing power of the brain is limited, it needs to be focused on task-relevant brain areas, with alpha putting the brake on task-irrelevant areas. When someones' eyes are closed, alpha power over the occipital part of their scalp is increased, as the visual cortex (located occipitally) is now task-irrelevant. Conversely, occipital alpha power decreases again when eyes are opened or, to a lesser extent, visual imagination is tasked. In other areas of the brain, alpha oscillations serve the same function, albeit specifics are dependent on what the respective brain region is doing for which alpha power levels are measured. For example, over the motor cortex a decrease in alpha oscillations (referred to as mu waves to make their separate origin clearer) is most pronounced when it is engaged during real or imagined movement, and an increase is most pronounced at rest.
Given that even in its early days, there was much speculation about the functional role of alpha oscillations, it didn't take long for researchers to wonder whether they could manipulate alpha power in human and non-human animals.
In 1958, Kamiya first showed that alpha power (now more broadly catalogued between 8-12 Hz) could be modulated at will. In his experiment (detailed in a 2011 paper cited in the bibliography of this article), an audio signal was triggered when the alpha levels of participants surpassed an arbitrary threshold. Focusing their attention on triggering the audio signal consciously, allowed participants to regulate their own brain activity. While they were not aware of what exact brain processes they were recruiting in order to trigger the audio signal, successfully turning it on was enough reward for the brain to let it know that it was doing a good job, creating a positive feedback loop.
In 1968, Wyrwicka and Sterman showed that this technique could be applied to non-human animals as well. They created a machine which automatically dispensed milk to cats once their sensorimotor rhythm (SMR) over their motor cortex had increased.
The Sensorimotor Rhythm
Likely unbeknownst to the cats themselves, as a consequence of the conditioning by Wyrwicka and Sterman, they cats changed their behaviour in order to maximise SMR rhythms (by regularly engaging in motor-relaxing movements like stretches, for example), which had neuroplastic effects of long-term increases in their SMR.
Sterman was pleased to see that cats could be conditioned to display enhanced SMR amplitudes, but as at that time, its inhibitory function was not yet understood, what happened next took him by surprise. One year later, he was tasked by the US Air Force to investigate the toxicity of rocket fuel for space travel (Sterman, LoPresti and Fairchild, 1969). As the rocket fuel contained compounds (monomethyl hydrazine – MMH) that disrupt the synthesis of inhibitory neurotransmitters, exposure to them meant runaway charges of brain activity, leading to seizures which ultimately would prove deadly. To test this, and with it minimise the risk for NASA's astronauts, Sterman administered increasing doses of MMH to the cats – including the ones whose SMR levels he had increased one year earlier. Curiously, only the ones with boosted SMR power survived seizure-free or required higher doses/longer exposure to show symptoms. Since, as explained, SMR increases the ability of the brain to coordinate motor action via inhibition, its heightened levels counter-acted the disinhibitory influence of the rocket fuels, making the cats perfectly resilient.
Sterman didn't just create "super cats", but also tested neurofeedback on patients suffering from epilepsy. Specifically, he set up two rows of lights which would light up in sequence as the SMR activity of the patient (as measured by EEG) increased, accompanied by a little chime as an additional reward signal, and a double chime upon completion of a row. This was repeated 100 times, both conditioning the patient and teaching them how to willingly increase SMR activity. As a result of the training, Sterman reports that patients significantly reduced their subsequent seizures (Sterman and Friar, 1972). Combined, this showed that this technique – if applied correctly – can change brain activity in a meaningful and long-lasting way.
Other more recent research by Ghaziri and colleagues has shown that neurofeedback can bring about successful enhancement of frontal beta 1 (12-20 Hz) power. As the frontal cortex is especially active during conscious reasoning and deliberate cognitive control, it is perhaps not surprising that enhancing its beta 1 activity via neurofeedback led to an increase of the potential to pay attention in participants (compared to non-intervention controls). Especially convincingly, the study was able to demonstrate that only neurofeedback conditions led to a long-term increases in grey- and white-matter volume in participants which accompanied the increase in beta 1 power (Ghaziri et al,. 2013). Similar long-term changes in EEG profiles and behavioural measures were found by a two-year follow-up study evaluating placebo-controlled neurofeedback treatment of ADHD by Becerra et al. (2006).
While this might give you hope that you could improve your brain function by just enhancing your alpha or beta activity, this is likely not so. In fact, just blindly increasing the levels of one frequency band is not necessarily going to do good. Brains are complicated, and what might help some could actively harm others. This is because research indicates that there are optimally stable levels of brain activity patterns and as your brain activity is so unique to you that an EEG frequency profile could identify you as readily as a fingerprint, that means taking your specific frequency profile into account first before deciding on a specific neuromodulatory protocol. Reaching ideal mental states is likely more about balancing activity in a highly personal manner than about blindly increasing it – especially as excessively strong alpha and beta oscillations are sometimes associated with cognitive and motor impairments.
Too high beta activity, for example, is often seen in Parkinson's disease (Tinkhauser et al., 2017), where the ability to voluntarily control body movements deteriorates, both in terms of involuntary shaking and stiffness in controlled motion. In such cases, it is interventions that reduce rather than increase beta power which help to alleviate negative symptoms. This is because the power of a frequency band reflects the degree of synchronisation of a network of neurons, which in turn reflects their network complexity. I wrote about this to a greater extent in my "Neural Oscillations" article, but to summarise one of its main points concisely, the brain maximises its computational potential by striking a balance between desynchronised and synchronised states of neuron activity. Too little synchronsiation and neuron firing is random, not allowing for complex computations. Too much synchronisation and activity overlaps, making it impossible for neurons to communicate effectively. Hence, while increasing beta activity via neurofeedback like it was done by Ghaziri and colleagues might be great for those who show less than average beta activity, it might be actively harmful for those with already normal or excessive levels of beta synchronisation.
This is important to point out also in a clinical context, where one might be intrigued by the idea of modulating brain activity in order to treat neuropathologies. Here too no fixed approach is likely to be ideal. Aberrations of brain activity in patients with ADHD or depression, for example, manifest in diverse ways even when behavioural symptoms might seem identical. Consequently, rather than enhancing or decreasing alpha or beta, the goal should be normalised brain activity in reference to a model of a healthy brain.
Neurofeedback Demo
What you can see in the video below is an implementation of such a brain-training mechanism I wrote in Matlab and Python. My brain signals are picked up by a custom EEG headset and then sent to my computer via Bluetooth. I then correct for artifacts and extract a frequency profile in real-time. In the script I wrote, I can then specify the frequency range I want to increase or decrease, and then observe colour changes in the blue square in the top-left corner, which grows lighter the closer I get to my desired mental state. In this way, by trying to consciously make the cube glow brighter, I can significantly change my brain activity in just a few minutes.
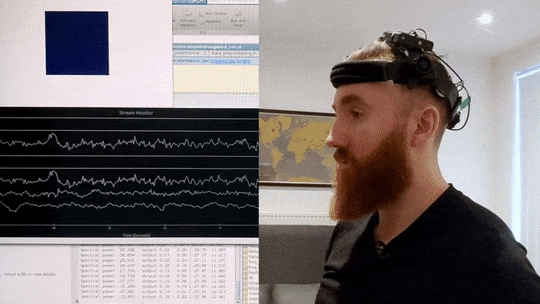
Real-time Neurofeedback Training
Visual demonstration of neurofeedback training system showing brain activity modulation in real-time
Heart Rate Variability Biofeedback
Homeostatis in the brain aside, in order to keep itself healthy and functional, the body at large employs a number of homeostatic mechanisms which keep its internal state approximately consistent. Since the contexts and environments that a person finds itself in change over time, some of that stability is achieved by processes of allostasis. Put simply, the body introduces chaotic fluctuations to homeostatic control mechanisms to make it more resilient. In slightly more technical terms, by introducing a noise signal that simulates changing conditions, the body is more able to better anticipate and deal with dyshomeostastic states that might result as a consequence of a volatile environment (McEwen, 1998).
As a consequence of introducing fluctuations to otherwise cyclical bodily processes, the body as a whole becomes more adaptive to changing environmental demands. A good example of this is the profile of a healthy heart. Rather than beating like a perfectly tuned metronome, it shows great variation in time intervals between individual heartbeats. In short, complexity/variability in beat-to-beat heart rate is a sign of healthy flexibility to adjust to both internal and external changes in demands and environments. This flexibility allows other parts of our body to keep fluctuations in check – allostasis in one part of the body allows for homeostasis in another. To give an example, fluctuations in heart rate rhythms enable keeping our blood pressure at rest within a stable range. Conversely to heart rate, variability in blood pressure is a sign of pathology, so this is critical to overall health and wellbeing.
Between individuals, their HRV gives some insight into their psychological and physiological health. For example, high HRV is associated with more successful emotion regulation (Geisler, Kubiak, Siewert, and Weber, 2013), superior cognitive abilities like information processing and executive functioning (Thayer et al., 2012) and general wellbeing (Mulcahy, Larsson, Garfinkel and Critchley, 2019). Conversely, low HRV correlates with increased morbidity and cardiac mortality (Huikuri, Perkiömäki, Maestri, and Pinna, 2009), a greater prevalence of depression and anxiety (Bos, Jentgens, Beckers, and Kindt, 2013), stress (Lischke et al., 2018) and a diminished ability to pay attention (Ramírez, Ortega, and Del Paso, 2015).
Basically, HRV is a snapshot of the autonomic nervous system (which controls everything that happens automatically in your body). Between individuals its variability reflects differences in more general cardiovascular health. HRV for any particular person is not static, however. Over a 24-hour period it changes primarily as a consequence of circadian rhythms and metabolisms. Over a short period, however, HRV is affected by more immediate things that happen in your body as a consequence of what you're doing and experiencing.
One factor contributing to HRV is blood pressure. It has its own endogenous signal in the body. Feedback oscillations in arterial blood pressure go up and down about six times per minute (or 0.1 Hz). Cardiopulmonary baroreceptors detect respiratory changes in cardiac filling and arterial pressure: when blood pressure goes down, our heart rate goes up, and vice versa.
The main source of HRV, however, is respiratory sinus arrhythmia (RSA) – a reflex from the brainstem to the heart. Essentially, heartbeat rhythms change in part due to changes in breathing. As lungs fill with more oxygen, the heart beats faster to deliver it to the brain and body more effectively. When oxygen is sparse, on the other hand, the heart slows down in order to not waste energy on unnecessary beats. Given that we have a finite number of heartbeats in life, it's nice to save a few.
This insight led Brown et al. (1993) to investigate which breathing rhythms would yield the biggest increase in HRV. After measuring HRV responses to different breathing speeds, they concluded that, for most people, these ideal rhythms lie somewhere between 6-10 breaths per minute, with the most pronounced average response at 6 breaths or 0.1 Hz (perhaps a consequence of resonance between the RSA and baroreceptors which also oscillate at around 0.1 Hz).
From here, biofeedback researcher picked up the torch, adding in a live measure of HRV that would guide the breathing tempo of the participant in real-time, as to most effectively maximise it. As a result, we have learned that HRV feedback and controlled slow-breathing can elicit increases in HRV (Lehrer et al., 2013; Lehrer & Gevirtz, 2014) and engender positive emotional effects, including significant reductions in self-reported anxiety and stress (Goessl, Curtiss, & Hofmann, 2017), post-stroke depression symptoms (Li et al., 2015), and post-traumatic stress disorder symptoms (Tan, Dao, Farmer, Sutherland, & Gevirtz, 2011).
HRV Biofeedback Demo
The video below shows me using a custom heart rate sensor, which, via an Arduino, directly connects to my computer. A program I wrote in Python then filters and pre-processes the signal before estimating the timing between individual beats with millisecond precision. Then, it calculates the variance in inter-beat intervals. Simultaneously, it displays an oscillating shape to guide my breathing, which changes its pacing in real-time in order to maximise said variance in time between individuals heart beats. If the code calculates that breathing slower has increased my HRV it will continue to instruct me to breathe at a progressively slower pace, up until this yields negative returns, and the pace is increased again. Once an optimal breathing rate is found, it is kept constant for 5 minutes, which significantly increases my HRV. In the video below, you can see my heart rate in the top left corner, changes in inter-beat intervals in the middle, and my increase in HRV in the top right corner. The oscillating circle that guides my breathing is displayed below.
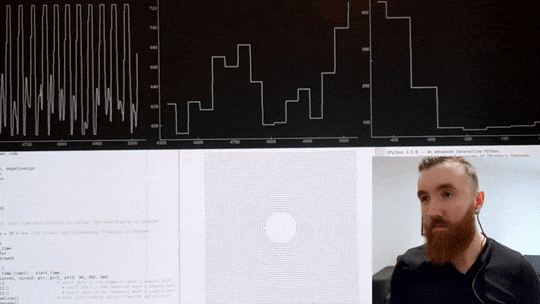
Heart Rate Variability Training
Real-time HRV biofeedback system showing heart rate monitoring and breathing guidance
I speculate that the positive effects of HRV biofeedback are all a consequence of resonant breathing putting additional stressors on the cardiovascular system, strengthening it via hormesis (positive adaptation to "low-dose" stressors) and hence improving overall wellbeing (Calabrese and Baldwin, 1998). In this sense, it would be not unlike how exercise puts stress on muscles, which strengthens them over time.
Future Directions
The idea of taking real-time sensor input in order to feed it to software which can then give the user personalised guidance is fascinating not just because of its potential to give greater insight and autonomy of psychological and biological processes of which we are normally completely unaware. Going beyond therapeutic – and possibly augmentative – uses of technology, the principle which underlies it can easily be applied to building more complete interactions between technology and humans.
Specifically, brain-computer interfaces can benefit from being able to enter into a dialogue with biology through real-time sensor feeds, in order to better accommodate for individual variation in neural makeup. For example, even now prosthetics can be controlled by neural activity generated by imagining the movement that the patient would like to perform. However, algorithms which interpret the neural signals often struggle with individual variation in the specific patterns of activity generated by said imagined motor actions, and patients sometimes have difficulty with conceptualising what exactly it means to imagine moving a non-existent limb with your mind. Biofeedback provides a solution to this. By feeding back a real-time visualisation of their brain activity to the participant, they are able to see which way of visualising the movement creates a more pronounced response, which significantly increases classification accuracy and reduces training time (Hwang, Kwon and Im, 2009).
Principles of biofeedback can also benefit effectiveness of brain stimulation technologies. By this I mean all systems that apply a force (perhaps electromagnetic pulses, as in transcranial magnetic stimulation) to the brain, in the hopes of it changing brain activity into a desired direction, usually for therapeutic applications or (clinical) study. Traditionally, brain stimulation has been "open loop", which applied some fixed stimulus to the brain in hopes of changing its activity in the desired direction. "Open loop", more generally, refers to non-feedback control systems where the output the control system achieves has no bearing on the input/control signal it emits. If the output an input creates is predictable this might be "good enough". For brain stimulation, however, it can, on theoretical grounds, not be up to the task. Specifically, this way of stimulating brains implies that there are little differences between people in terms of neural makeup, so that the same stimulation approach would work across otherwise diverse populations, and secondly that the brain response to exogenous intervention will be mostly linear (the assumption that, say, for any kind of rhythmic brain stimulation, a stimulation frequency applied will equal the stimulation frequency induced) and hence predictable, with no change in response over time or depending on what else is happening in the brain while the stimulation is applied. Both of these assumptions are untenable. Firstly, we know that even within roughly homogeneous populations, for example within children diagnosed with ADHD, the neural substrate which gives rise to almost identical behavioural symptoms is heterogeneous (Arns, Conners and Kraemer, 2013). Secondly, we know that even basic neural mechanisms, such as the firing of a single neuron, are non-linear (Hodgkin and Huxley, 1952). With the help of biofeedback, the "open loop" can be closed. In closed-loop stimulation, as opposed to open-loop approaches, the output the control system generates immediately adjusts the input/control signal, so rather than blindly stimulating, the stimulation is adjusted in order to account for individual differences in neural response to the stimulation. This has the promise of both being a safer and more effective means of neuromodulation, and it is one of the central focus points of my research.
Biofeedback, especially defined in these broader terms, brings a number of fascinating concepts to the table, which I think researchers and engineers in many disciplines will find value in. Whether it's for psychological or physical improvement (imagine, for example, a smart sensor that gives real-time feedback on exercise routines and forms), more advanced human-computer interactions or personalised forms of brain-stimulation, biofeedback holds potential for allowing us to establish a more symbiotic relationship between biology and technology. Let's apply some of its principles to help improve lives!
Sources
Arns, M., Conners, C.K. and Kraemer, H.C., 2013. A decade of EEG theta/beta ratio research in ADHD: a meta-analysis. Journal of Attention Disorders, 17(5), pp.374-383.
Becerra, J., Fernandez, T., Harmony, T., Caballero, M.I., Garcia, F., Fernandez-Bouzas, A., Santiago-Rodríguez, E. and Prado-Alcalá, R.A., 2006. Follow-up study of learning-disabled children treated with neurofeedback or placebo. Clinical EEG and Neuroscience, 37(3), pp.198-203.
Berger, H., 1929. Über das Elektroenkephalogramm des Menschen. Archiv für Psychiatrie und Nervenkrankheiten, 87(1), pp.527-570.
Bos, M.G., Jentgens, P., Beckers, T. and Kindt, M., 2013. Psychophysiological response patterns to affective film stimuli. PloS One, 8(4), p.e62661.
Brown, T.E., Beightol, L.A., Koh, J. and Eckberg, D.L., 1993. Important influence of respiration on human RR interval power spectra is largely ignored. Journal of Applied Physiology, 75(5), pp.2310-2317.
Calabrese, E.J. and Baldwin, L.A., 1998. Hormesis as a biological hypothesis. Environmental Health Perspectives, 106(suppl 1), pp.357-362.
Geisler, F.C., Kubiak, T., Siewert, K. and Weber, H., 2013. Cardiac vagal tone is associated with social engagement and self-regulation. Biological Psychology, 93(2), pp.279-286.
Ghaziri, J., Tucholka, A., Larue, V., Blanchette-Sylvestre, M., Reyburn, G., Gilbert, G., Lévesque, J. and Beauregard, M., 2013. Neurofeedback training induces changes in white and gray matter. Clinical EEG and Neuroscience, 44(4), pp.265-272.
Hodgkin, A.L. and Huxley, A.F., 1952. A quantitative description of membrane current and its application to conduction and excitation in nerve. The Journal of Physiology, 117(4), pp.500-544.
Huikuri, H.V., Perkiömäki, J.S., Maestri, R. and Pinna, G.D., 2009. Clinical impact of evaluation of cardiovascular control by novel methods of heart rate dynamics. Philosophical Transactions of the Royal Society A: Mathematical, Physical and Engineering Sciences, 367(1892), pp.1223-1238.
Hwang, H.J., Kwon, K. and Im, C.H., 2009. Neurofeedback-based motor imagery training for brain–computer interface (BCI). Journal of Neuroscience Methods, 179(1), pp.150-156.
Kamiya, J., 2011. The first communications about operant conditioning of the EEG. Journal of Neurotherapy, 15(1), pp.65-73.
Sterman, M.B., LoPresti, R.W. and Fairchild, M.D., 1969. Electroencephalographic and behavioral studies of monomethylhydrazine toxicity in the cat. California Univ Los Angeles Brain Research Inst.
Sterman, M.B. and Friar, L., 1972. Suppression of seizures in an epileptic following sensorimotor EEG feedback training. Electroencephalography and Clinical Neurophysiology, 33(1), pp.89-95.
Tinkhauser, G., Pogosyan, A., Tan, H., Herz, D.M., Kühn, A.A. and Brown, P., 2017. Beta burst dynamics in Parkinson's disease OFF and ON dopaminergic medication. Brain, 140(11), pp.2968-2981.
Wyrwicka, W. and Sterman, M.B., 1968. Instrumental conditioning of sensorimotor cortex EEG spindles in the waking cat. Physiology & Behavior, 3(5), pp.703-707.